Why Diagnostic Process Mining is Like Detective Work
- Niyi Ogunbiyi
- Feb 8
- 3 min read
Updated: Feb 9
In recent years, the field of process mining has seen a surge in interest, particularly in descriptive process mining techniques like process discovery, which involves using algorithms to uncover the true nature of business processes from event logs. This approach provides valuable insights into what is happening within an organization. However, while these descriptive methods can highlight issues such as high levels of rework, bottlenecks causing delays, or instances missing deadlines, they often fall short in explaining why these problems occur.
To understand the true underlying causes of these issues, we require diagnostic process mining. This set of tools and techniques aims to identify the root causes of process inefficiencies, enabling organizations to implement effective solutions. Understanding the true causes is crucial; without this knowledge, any corrective actions may consume time and resources without effectively resolving the problem.
Consider a scenario where an organization is grappling with a lower-than-expected On-Time Delivery (OTD) rate for cases. Upon examining a typical root cause analysis dashboard, various metrics are displayed, including average delay and OTD rates across different teams (see Fig 1 below).

Suppose the data indicates that 'Team Hermes' has a lower OTD rate and higher average delay compared to other teams. At first glance, one might conclude that the team's performance issues are due to internal factors, such as team members not meeting expectations or lax management. Consequently, stricter oversight might be implemented.
However, this conclusion is based on observed associations and may overlook confounding factors. In this case, a critical confounder could be case complexity. If 'Team Hermes' is assigned more complex cases, which are inherently more prone to delays, the team's lower OTD rate is not due to internal shortcomings but rather the nature of their assignments. Without recognizing this, implemented solutions not only fail to improve the OTD rate but may also demoralize team members and increase attrition.
Currently, only a few process mining vendors offer causal inference tools that can identify such confounding factors. Many existing root cause analysis dashboards display associational rather than causal relationships, which can lead to misguided conclusions.
Performing causal analysis in process mining is akin to a detective investigating a crime. The objective is to determine who committed the crime and, if multiple parties are involved, their degree of involvement. The detective gathers evidence from various sources—CCTV footage, interviews, phone records—and pieces together a case.
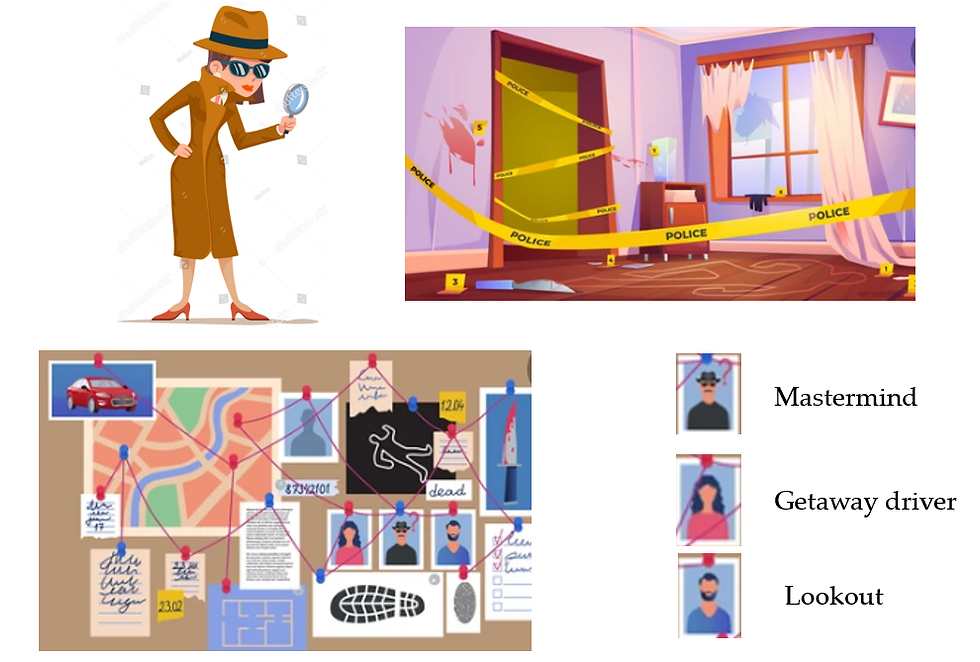
Similarly, in diagnostic process mining, we collect evidence from event logs, analyze the data, consult with subject matter experts, and apply inductive and deductive reasoning, along with causal inference techniques, to identify the causes of process issues and assess their impact. This approach also allows us to evaluate the effect of potential solutions and determine which subsets of cases to target with interventions.
In this series of blog posts, we will embark on an end-to-end journey using a real-life event log to explore diagnostic process mining. The series will cover the following topics:
Defining the Problem: How to clearly articulate the process issue at hand.
Identifying Causes and Estimating Their Impact: Techniques to uncover root causes and assess their contribution to the problem.
Validating the Causes: Methods to confirm that the identified causes are indeed responsible for the observed issues.
Finding Solutions for the Identified Causes: Developing effective interventions to address the root causes.
Validating the Solutions: Ensuring that the implemented solutions effectively resolve the problem.
Prescriptive Process Monitoring Solutions: Discussing approaches to monitor processes proactively and prescribe actions to prevent future issues.
Our aim is to make these posts accessible to all readers by prioritizing intuition and comprehension. We will focus on explaining the results in an easily understood manner, without delving into the mathematical foundations of causal inference techniques.
We hope you will join us in the next post as we begin this insightful journey into diagnostic process mining.
Comentários